PREDICTION OF DOXYCYCLINE REMOVAL BY PHOTO-FENTON PROCESS USING AN ARTIFICIAL NEURAL NETWORK - MULTILAYER PERCEPTRON MODELtificial neural network - multilayer perceptron model
Original scientific paper
DOI:
https://doi.org/10.2298/CICEQ230824009BKeywords:
Doxycycline hydrate, modelling, photo-fenton, optimized artificial neural network, removalAbstract
This paper presents a study on the effectiveness of the Photo-Fenton Process (PF) for removing the doxycycline hyclate (DXC) antibiotic. The experiment showed that the best removal efficiency was achieved (79%) at pH 3 for 2.5 mg/L of DXC, 76.53 mg/L of H2O2, and 86.8 mg/L of Fe2+. The degradation mechanism of DXC by hydroxyl radicals was confirmed by FTIR and HPLC. To model the oxidation reaction of DXC by PF, a multilayer perceptron (MLP) based optimized artificial neural network (OANN) was used, taking into account experimental data such as pH and initial concentrations of DXC, H2O2, and Fe2+. The OANN predicted removal efficiency results were in close agreement with experimental results, with an RMSE of 0.0661 and an R2 value of 0.99998. The sensitivity analysis revealed that all studied inputs significantly impacted the transformation of DXC.
References
[1] A. A. Borghi, M. F. Silva, S. Al Arni, A. Converti, and M. S. A. Palma, J. Chem. 2015 (2015) 1—9. https://doi.org/10.1155/2015/492030.
[2] D. Xu Y. Gao, Z. Lin, W. Gao, H. Zhang, K. Karnowo, X. Hu, H. Sun, S. Shatir, S. Hassan, S. Zhang, Front. Chem. 7 (2020) 1—18. https://doi.org/10.3389/fchem.2019.00943.
[3] J. Scaria, K. V. Anupama, P. V. Nidheesh, Sci. Total Environ. 771 (2021) 145291. https://doi.org/10.1016/j.scitotenv.2021.
[4] R. Daghrir, P. Drogui, Environ. Chem. Lett. 11 (2013) 151—156. https://doi.org/10.1007/s10311-012-0390-2.
[5] T. Han, Y. Liang, Z. Wu, L. Zhang, Z. Liu, Q. Li, X. Chen, W. Guo, L. Jiang, F. Pan, S. Ge, Z. Mi, Z. Liu, H. Huang, X. Li, J. Zhou, Y. Li, J. Wang, Z. Zhang, Y. Tang, M. Wu, J. Hazard. Mater. 380 (2019) 120885. https://doi.org/10.1016/j.jhazmat.2019.120885.
[6] J. Bolobajev, M. Trapido, and A. Goi, Chemosphere 153 (2016) 20—23. http://doi.org/10.1016/j.chemosphere.2016.03.042.
[7] M. Hui Zhang, H. Dong, L. Zhao, D. X. Wang, D. Meng, Sci. Total Environ. 670 (2019) 11—21. https://doi.org/10.1016/j.scitotenv.2019.03.180.
[8] P. V. Nidheesh, R. Gandhimathi, and S. T. Ramesh, Environ. Sci. Pollut. Res. 20 (2013) 2099—2132. https://doi.org/10.1007/s11356-012-1385-z.
[9] S. M. Aramyan, , Int. J. Environ. Sci. Nat. Resour. 2 (2017) 1—18. ISSN: 2572-1119.
[10] Y. Mesellem, A. A. El Hadj, M. Laidi, S. Hanini, M. Hentabli, Neural Comput. Appl 2 (2021). https://doi.org/10.1007/s00521-021-05890-2.
[11] A. Adda, S. Hanini, S. Bezari, M. Laidi, M. Abbas, Environ. Eng. Res. 27 (2021). https://doi.org/10.4491/eer.2020.383.
[12] P. Kundu, A. Debsarkar, S. Mukherjee, S. Kumar, Environ. Technol. (United Kingdom). 35 (2014) 1296—1306. https://doi.org/10.1080/09593330.2013.866698.
[13] A. Giwa, A. Yusuf, H.A. Balogun, N.S. Sambudi, M.R. Bilad, I. Adeyemi, S. Chakraborty, S. Curcio, Process Saf. Environ. Prot. 146 (2021) 220—256. https://doi.org/10.1016/j.psep.2020.08.015.
[14] O. B. Ayodele, H. S. Auta, N. Md Nor, Ind. Eng. Chem.
Res. 51 (2012) 1611—1619. https://doi.org/10.1021/ie302390b.
[15] S. Talwar, A. K. Verma, V. K. Sangal, J. Environ. Manage., 250, July, (2019), https://doi.org/10.1016/j.jenvman.2019.109428.
[16] S. Sethi, A. Dhir, V. Arora, React. Kinet. Mech. Catal., 136, 1, (2023) 549—565, https://doi.org/10.1007/s11144-023-02360-9.
[17] N. Boucherit, M. Abouseoud, L. Adour, Nov. Biotechnol. Chim. 17 (2018) 160—171. https://doi.org/10.2478/nbec-2018-0017.
[18] A. Babuponnusami, K. Muthukumar, J. Environ. Chem. Eng. 2 (2014) 220—226. http://doi.org/10.1016/j.jece.2013.10.011.
[19] L.G. Devi, K.E. Rajashekhar, K.S. Anantha Raju, S.G. Kumar, Desalination 270 (2011) 31—39. http://doi.org/10.1016/j.desal.2010.11.017.
[20] J. Jeong, W. Song, W. J. Cooper, J. Jung, J. Greaves, Chemosphere 78 (2010) 535—540. http://doi.org/10.1016/j.chemosphere.2009.11.024.
[21] R. Pulicharla S.K. Brar, T. Rouissi, S. Auger, P. Drogui, M. Verma, R.Y. Surampalli, Ultrason. Sonochem. 34 (2017) 332—342. http://doi.org/10.1016/j.ultsonch.2016.05.042.
[22] E. S. Elmolla, M. Chaudhuri, and M. M. Eltoukhy, J. Hazard. Mater. 179 (2010) 127—134. http://doi.org/10.1016/j.jhazmat.2010.02.068.
[23] M. Laidi and S. Hanini, Int. J. Refrig. 36 (2013) 247—257. http://doi.org/10.1016/j.ijrefrig.2012.09.016.
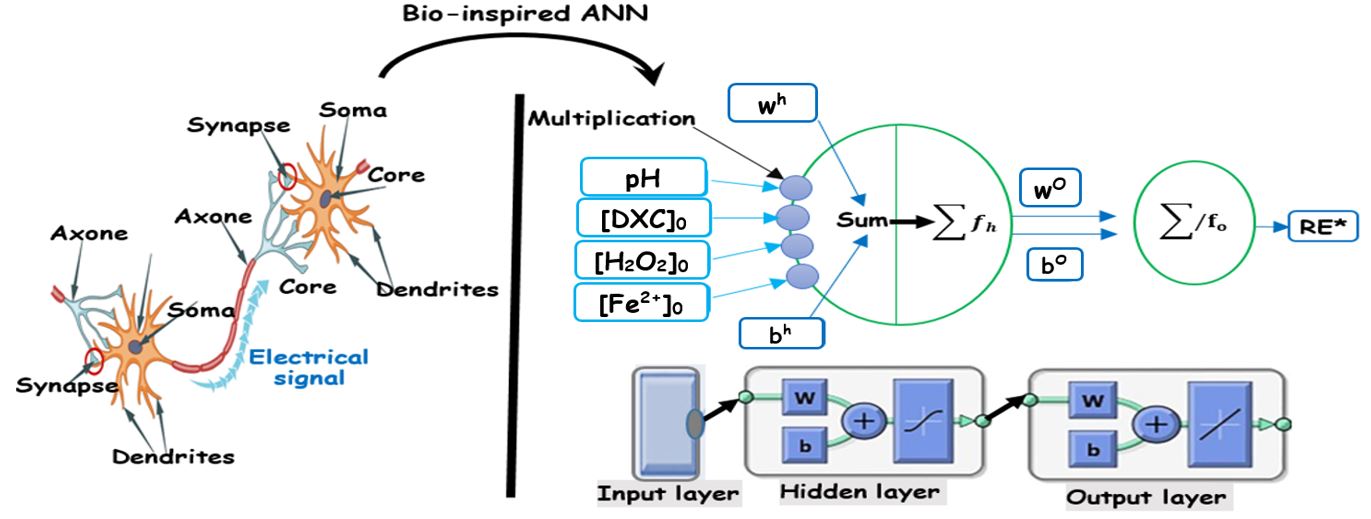
Downloads
Published
Issue
Section
License
Copyright (c) 2023 Nabila Boucherit, Salah Hanini, Abdellah Ibrir, Maamar Laidi, Mohamed Roubehie Fissa

This work is licensed under a Creative Commons Attribution-NonCommercial-NoDerivatives 4.0 International License.
Authors who publish with this journal agree to the following terms:
Authors retain copyright and grant the journal right of first publication with the work simultaneously licensed under a Creative Commons Attribution License that allows others to share the work with an acknowledgement of the work's authorship and initial publication in this journal.
Authors grant to the Publisher the following rights to the manuscript, including any supplemental material, and any parts, extracts or elements thereof:
- the right to reproduce and distribute the Manuscript in printed form, including print-on-demand;
- the right to produce prepublications, reprints, and special editions of the Manuscript;
- the right to translate the Manuscript into other languages;
- the right to reproduce the Manuscript using photomechanical or similar means including, but not limited to photocopy, and the right to distribute these reproductions;
- the right to reproduce and distribute the Manuscript electronically or optically on any and all data carriers or storage media – especially in machine readable/digitalized form on data carriers such as hard drive, CD-Rom, DVD, Blu-ray Disc (BD), Mini-Disk, data tape – and the right to reproduce and distribute the Article via these data carriers;
- the right to store the Manuscript in databases, including online databases, and the right of transmission of the Manuscript in all technical systems and modes;
- the right to make the Manuscript available to the public or to closed user groups on individual demand, for use on monitors or other readers (including e-books), and in printable form for the user, either via the internet, other online services, or via internal or external networks.